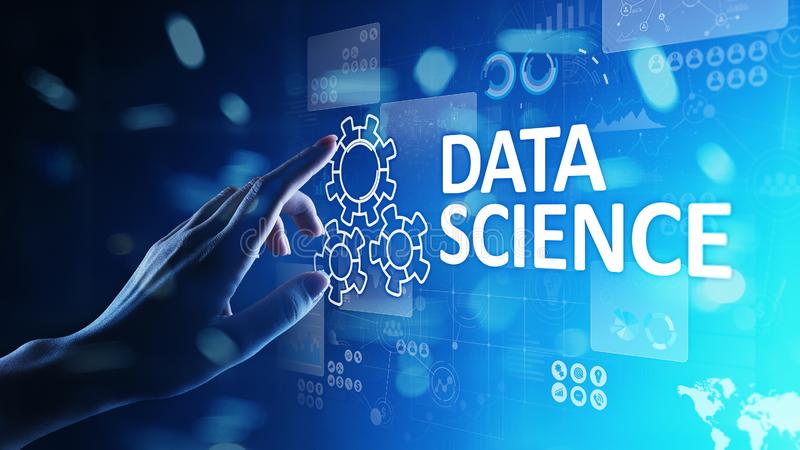
With the rise of Big Data management concepts, we are seeing rapid adoption of data science techniques to retrieve vital information from unstructured data sources. These projects are mostly led by trained data analysts and IT managers who have recognized data science certification from top institutes in India.
When we talk about data, we are often struck with the various nuances of data operations (popularly referred to as DataOps) which could be related to any of the following sub-fields mentioned below:
- Data Management
- Data Storage
- Data Intelligence
- Data Analytics
- Data Repurposing, and so on
In general terms, we are evaluating mostly the role of analytics in data storage techniques, and how data science practices influence key benchmarks or KPIs in the data management cycle. We still need highly trained and reliable ML models to identify the different types of data storage techniques and the activities that could simply data engineering as a whole. You would mostly work with three types of data storage practices;
- Auto-Search
- Document-based
- Object-based
In recent times, 90% of the data storage techniques are developed to handle rich metadata and parallel architecture that infinitesimally influence the way AI ML workflows are managed, allowing databases to grow continuously in scale, reliability, and agility.
Common techniques in data storage
Data storage management incorporates the following techniques that can be learned and experimented with, in a data science online course:
- Cloud computing
- Inferential statistics / computational statistics
- Statistical modeling
- Signal processing
- Natural Language Processing (NLP)
- Predictive Analytics
- Data extraction, loading, and transformation
Let’s understand the role of data science in Data Storage, as witnessed from recent developments and innovations in the industry.
Why you should learn about data storage management in a data science certification course
For top-grade data engineers, understanding how data storage works are critical to the success of many underlying practices that go into the development of modern IT frameworks, mostly supported by Cloud environments, AI Ops, and AutoML techniques. Newer concepts such as Dockers/ Kubernetes, containerization, virtualization, GPUs, and ETL techniques are related to data engineering, data storage, and retrieval – all put together in one basket. In data science certification, you would come across many topics related to search engines, content discovery, and document storage, and these can be understood by working in environments familiarized with SQL databases, Elasticsearch, Redshift, MongoDB, Apache Kafka, AWS, PostgreSQL, and so on. A solid understanding of Python and R programming languages often comes in handy in dealing with graphical databases and knowledge lakes that are built and designed as per modern demands of data storage requirements.
Data engineers have to embrace data science to handle these massive volumes of data supported on traditional infrastructure.