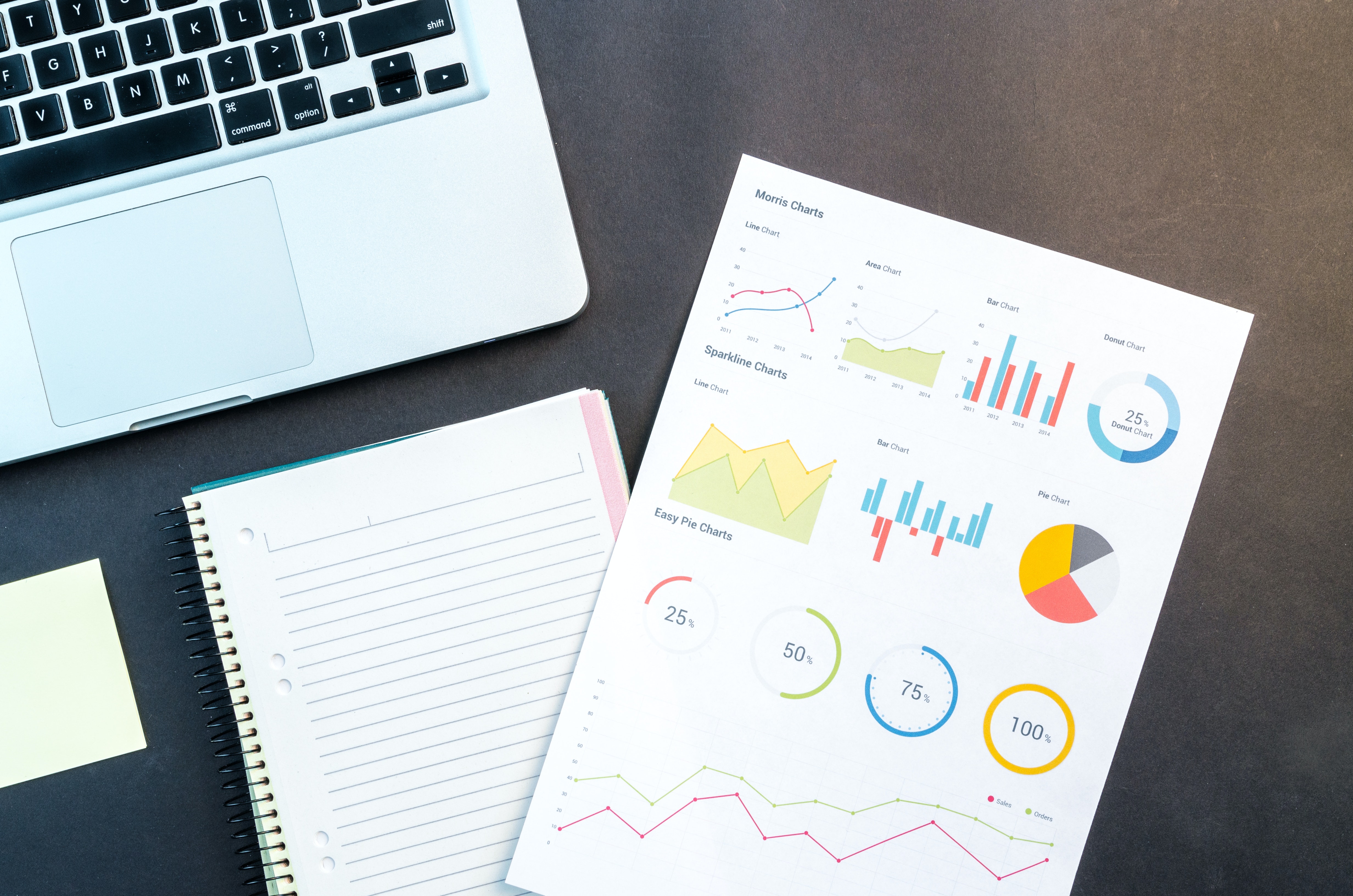
There are numerous reasons for bias in system studying packages. As statistics scientists, it's a part of our activity to do our first-rate to lessen and save you the reasons of bias of their fashions. The first-rate manner to save your bias is to recognize its reason completely. Once the reason has been identified, a few moves may be taken to do away with it and get a data science online course of its consequences entirely. This article will undergo the five important varieties of system studying bias, why they occur, and the way to lessen their effect.
№1: Algorithmic Bias
If your set of rules offers exclusive effects for nearly the same cases, then perhaps you want to get again and recheck your set of rules and if it's great in shape for the trouble at hand. Algorithmic bias can be both intentional and unintentional. It may be the result of technical problems withinside the middle of the set of rules or a wrongful desire of algorithms withinside the first place.
№2: Sample Bias
Another reason for bias in system studying packages is pattern bias. This form of data science course fees effects by a mistake withinside the early ranges of software improvement, that is the gathering and cleansing of statistics. Data is the middle of any system data science course in India software; after all, the set of rules can’t research what it didn’t see. Luckily, pattern bias isn't always that complicated to fix; you may binary multiplication the usage of larger, greater numerous datasets to educate your version. You can educate it a couple of times, look at its behavior, and finetune the parameters to attain the first-rate answer.
№3: Prejudice bias
You would possibly have an appropriate set of rules to your trouble, and you probably did your first-rate to select the first-rate pattern of statistics you may get, however still, your effects are biased. One cause that might appear is prejudice bias. Prejudice bias is frequently the result of the statistics being biased withinside the first place. The statistics you extracted and used to educate your version might also additionally have preexisting bais, including stereotypes and defective case assumptions. So, the usage of these statistics will continually bring about biased effects regardless of what set of rules you try and use. Prejudice bias is pretty hard to solve; you may try and use a wholly new dataset, try and adjust the statistics to do away with any present biases.
№4: Measurement Bias
Measurement bias might be the form of bias that happens very early in probability prediction, the improvement procedure, the statistics series level of the procedure. If the statistics that the version's overall performance and accuracy completely rely on are faulty, not anything withinside the procedure's last steps could be. If those computational measurements are defective, they'll bring about misguided statistics factors affecting a good way to be fed to the version to educate it and broaden it.
№five: Exclusion Bias
Choosing an appropriate dataset to educate and construct your version isn't always a smooth task. One assignment that you could face whilst doing so is attempting to keep away from exclusion bias. Exclusion bias takes place while essential statistics factors are excluded from the education dataset, and consequently, the resulting version doesn't recollect them.
Takeaways
Both people and algorithms are mistakes-inclined and supervised learning. However, that doesn't imply that our fashions want to be biased as well. The generation around us is making maximum choices for us, choices on what to shop for subsequent, what faculty is higher for our kids, what town is more secure to transport into, whether or not or now no longer our subsequent mortgage request is approved, and lots of much greater. These systems, however, may be biased relying upon the statistics used to construct them and the character constructing them as well. That's why it's an important step of any system studying software improvement to try and lessen and do away with bias as much as we can. And to efficiently do that, we want to recognize why bias happens withinside the first place, its types, and in which every kind happens withinside the improvement procedure.