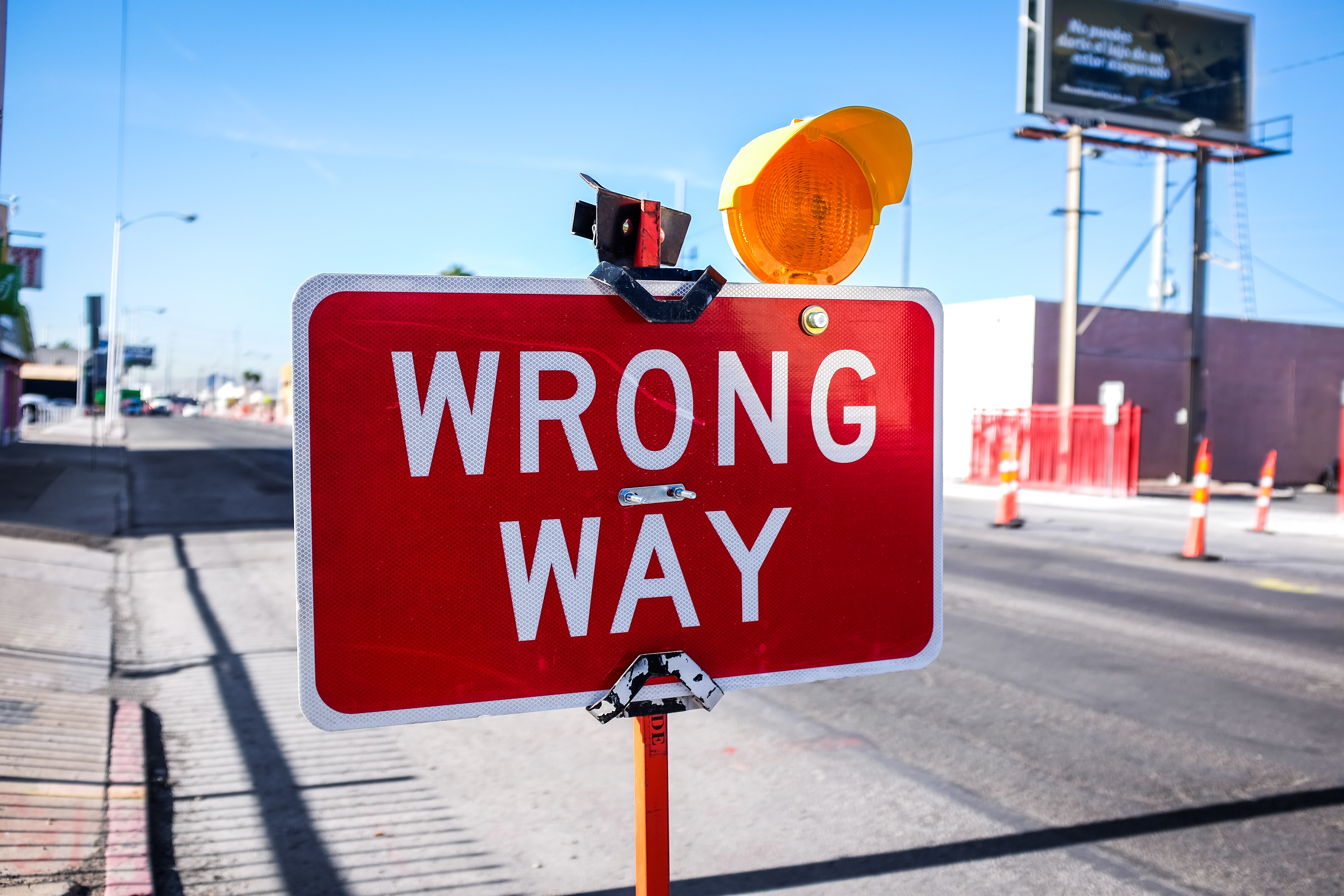
You've chosen data science, but learning it may be scary. Especially towards the beginning. As more companies become data-driven and the globe becomes more linked, every firm may require data science for representation. So, data positions are in high demand.
This blog discusses deadly data science blunders individuals make while beginning their professions. Mistakes may waste your time, energy, and enthusiasm if you're not cautious. By avoiding these blunders, you'll save days, weeks, or even months of aggravation. Let's get into our material.
Reputed institutes now offer the best online data science courses.
Data Science Rookie Mistakes
1. Start Enthusiastic, Get Jaded
Most individuals start their jobs with vigour. Data Science is a competitive and multifaceted discipline. Many data science experts struggle to continue their learning path. Anxiety is understandable since what you learn as a student and as a professional are quite different. Instead of worrying, concentrate on studying and working as much as possible since your experience will drive your career. What to do? Data Science Communities When you're unmotivated, do this. When you join this area, you discover how much people want to share ideas and expertise.
Beginning your education is vital to your long-term success. The decisions you take in the first few weeks will shape your habits and how you handle problems throughout your trip.
2. Theory-Heavy
Many newcomers waste too much time on theory, whether it's linear algebra, statistics, or machine learning algorithms, derivations, etc. Data science is an applied field; exercising builds skills. If you don't see the real application of what you're studying to the actual world, you may quit.
These theoretical techniques are taught in academics, but practitioners might benefit from a results-oriented perspective. Balance your academics with hands-on initiatives. Learn to be content with limited dimensional knowledge since you won't always comprehend everything, but it may help you later. Learn how each component fits into the overall picture with patience. You'll not only master theory, but also apply it in practice.
3. Skipping the Prerequisites for Machine or Deep Learning
Videos of robots, fantastic prediction models, and big pay encourage most people to become data scientists/analysts. But it's a long way off. Data job responsibilities are like a house's brick-by-brick construction.
Before applying approaches to a problem, learn the fundamentals. This will assist you learn how to fine-tune an algorithm and expand on current strategies. Math is vital, thus it helps to understand certain ideas. Master the basics. You should learn to swim like every Olympic diver.
4. Not Developing Data Visualisation Skills Early
Most data science beginners develop models and make predictions. Model construction is just 10% of the data science life cycle. Whether a data analyst or data scientist, everyone deals with data. Data scientists should have great data visualisation abilities. It helps create intuition and find data trends. This intuitive knowledge improves feature engineering, model building, and feature selection. How to improve?
- Learn data storytelling and dashboard development.
- Sample data may help you improve.
- Realize a project using these talents and get comments.
Most individuals stop learning after graphing using matplotlib or seaborn. Several institutes now offer the machine learning course online as well.
5. Only Using Certifications
Data science certificates and degrees are ubiquitous since they became popular. Too many data aspirants are taking these online courses. Hiring managers don't care much about paper credentials; they care more about your expertise and how you've used it in real-life scenarios.
Certifications are important, but not for the certificate itself, but for the abilities you learn. Interviews are key. The interviewer will test your talents. If you want a data science certification, stay up with your training and obtain the proper abilities. Skills, not a degree, get the job.
6. Guidance
Data Science, machine learning, and data engineering are emerging fields, as are its graduates. Few have cracked this code. The easiest method to join data science is to pay millions of rupees for a recognised certification, only to grow dissatisfied with the recorded videos or a YouTube playlist and not be industry-ready.
Find a data science mentor and ask how they handled their career, how they got the position they wanted, what resources they had, etc. Now, the last, often-overlooked component.
7. Miscommunication
As a data scientist, presenting your results to a business stakeholder is part of your work. Being able to pivot from a technical discourse to showcasing a commercial value in human words is important. You'll always have a corporate sponsor. They're not technical and won't join your team. They just care about themselves. Here comes your communication skills. These abilities can help you build business relationships and ace interviews. Communication is an undervalued ability.
You can now learn the best machine learning course online too.