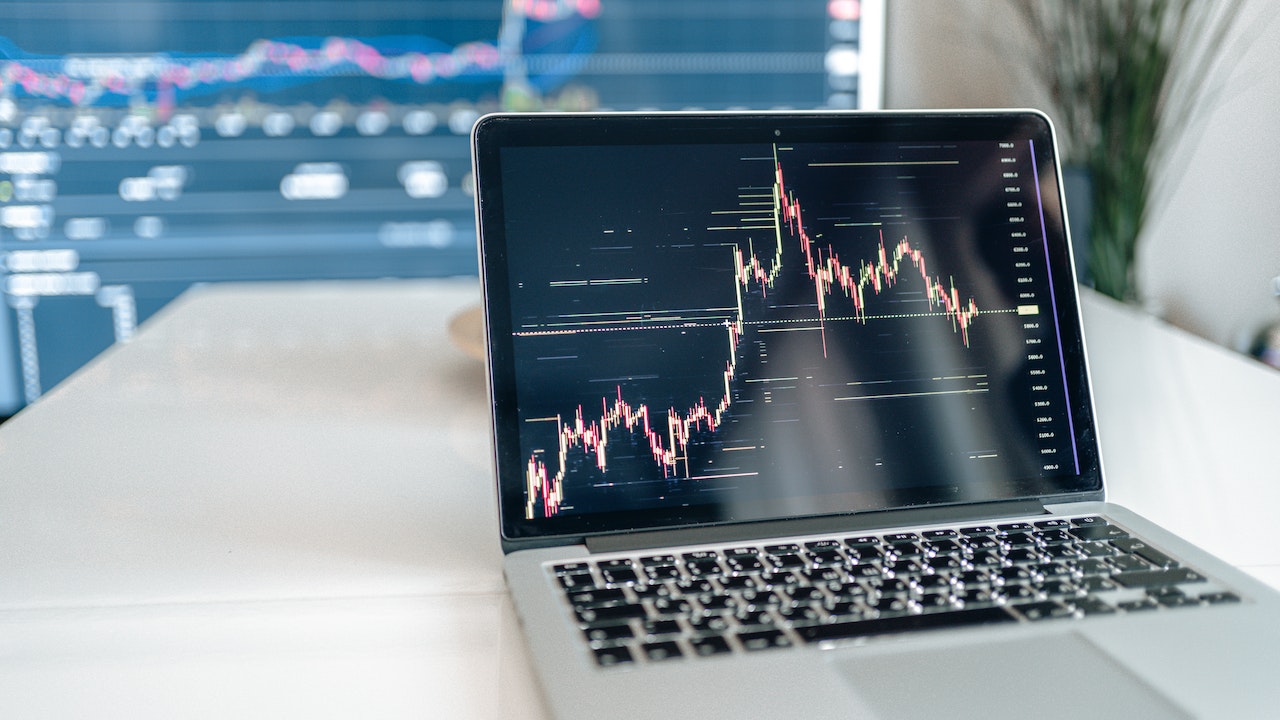
Introduction of Commodity Price Forecasting using AI/ML:
Price forecasting is the prediction of a resource or commodity price based on different criteria such as its qualities, demand, forecasts, the pricing of othear complementary or competitor commodities, proposals from various providers, and so on. It depends on various factors and the prices for the commodities fluctuate on a constant scale.
Why Price Forecasting?
Price forecasting might be a component of customer travel apps like Subway line or Hopper, which are designed to foster client loyalty and engagement. At the same time, other firms may benefit from price forecasting information. Businesses may need to determine the best moment to acquire a commodity to change the prices of goods and activities that need a commodity (lumber, coffee, gold), or to assess the financial appeal of fixed assets.
Commodity price forecasting is important in the strategic purchase and pricing of products that use these resources as raw materials. Forecasting enables planners to make data-driven choices, eliminate valuation risks, and integrate platforms proactively while avoiding procurement disruption due to price fluctuation.
Regression Analysis:
Regression analysis is a mathematical approach used to assess the connection between dependent and independent variables (such as the price of energy, a trip, or property) and one or more independent (interdependent) factors, often known as predictors, that influence the target variable. Researchers can also use regression analysis to discover how much these factors impact a target variable. A parameter in regression is always numerical.
Stock Price Prediction using Machine learning:
Shares Pricing using Deep Learning assists businesses in determining the future worth of a company's stock and other bank deposits transacted on a market. The main point of forecasting stock prices is to make large gains. It is difficult to predict how the stock market will fare. Other elements that influence prediction include physical and psychological aspects, rational and illogical conduct, and so on. All of these elements work together to make stock values dynamic and volatile. This makes accurate stock price forecasting extremely challenging.
Types of Price Forecasting Analytics:
Two types of analytics can be broadly used which include Predictive and Descriptive analytics.
Descriptive Analytics:
Analytics that are descriptive. Descriptive analytics is based on statistical approaches such as data gathering, analysis, interpretation, and presenting of results. Descriptive analytics enable the transformation of raw observations into knowledge that can be understood and shared. In a nutshell, this analytics category aids in answering the question, "What happened?" and gives output accordingly.
Predictive Analytics:
Analytics that predict. In the context of price forecasts, predictive analytics entails examining current and historical data to anticipate the likelihood of future occurrences, outcomes, or values. Many statistical approaches, such as data mining (finding patterns in data) and machine learning, are required for predictive analytics.
Benefits of Commodity price forecasting:
Future Demand Prediction:
In a few industrial businesses, demand is heavily influenced by raw material pricing, which aids in forecasting future demand.
Pricing of completed goods:
Raw material price forecasting is critical to product pricing and competitive dynamics. The final price point is generally some element of the cost of raw materials in the semi-finished goods industry.
Challenges of Commodity Price Forecasting:
Predicting the price of a product is a difficult task since relatively identical items with minor changes such as different brand names, extra specs, quality, demand for the product, and so on can have widely disparate costs.
We need a yardstick to determine how well or poorly our model performs. This is referred to as a yardstick performance measure or simply a metric in machine learning terms. A regression model's performance may be measured using a variety of measures, such as squared error, mean absolute error, and so on.
Supply Chain Challenges:
Supply chain disruptions induced by the coronavirus epidemic have created extraordinary price volatility in recent months, testing the discipline of commodity price forecasting to its very core. As a result, our team continues to collaborate with our customers to provide real-time pricing monitoring and tracking across regions based on macro trends such as global pandemic developments, border closures, labour market halts, and new laws, rules, and policies.
Fluctuations in market trends and prices:
The majority of regional and worldwide stainless-steel firms will have smaller profit margins. A sharp rise in crude oil market prices, as predicted by SpendEdge's most recent oil price estimate for 2019, would drive up utility expenses, especially those for gasoline and lubricants. Utility price increases will push up manufacturing and shipping costs for stainless steel firms. As a result of the increase in the crude oil market price, they will be forced to raise the pricing of final goods to cover their production costs.
Need for Artificial Intelligence-based forecasting:
Traditional forecasting methods are either both quantitative and qualitative analyses of the supply and demand sector indicators or statistical models employing univariate methodologies that depend solely on past price data. These approaches, however, fail to capture the whole market dynamics and do not work well for longer time horizon forecasting (weekly/monthly). AI/ML-based forecasting models, on the other hand, can deliver more accurate predictions over a longer time horizon. These algorithms can also analyze enormous amounts of historical data and uncover hidden trends to assist businesses in making better decisions.
Artificial Intelligence and Machine Learning in Price Forecasting:
Forecasting commodity prices is a critical procedure for a wide range of organizations. Price forecasting decisions have a direct impact on a company's bottom line and influence anything from partnerships to business planning. As a result, it is a field that needs extremely accurate insights and knowledge.
There are several levers and measures to examine when it comes to essential commodities like crude oil or gold. On paper, this makes them an excellent use case for narrow AI - an analysis job that requires a significant amount of manual labour and includes the processing of massive amounts of data.
Volatility issues of Commodities:
Certain commodities, where data quantities are lower and the link between the commodity and its drivers is not necessarily linear, might benefit from AI approaches to uncover hidden patterns or linkages and provide reliable forecasts.
The second significant reason is that many consumer products clients want to improve in this area. We were able to look at these concepts and abilities through the lens of their organization, elevating our work from academic exercises to actual, practical adjustments to assist manage crude price volatility in a real business context by collaborating with them.
Commodity price volatility worsens poverty and, in the worst-case scenario, is disastrous for thousands of millions of individuals affected by food insecurity, resulting in significant increases in famine and child mortality. Volatile prices of commodities, according to MIT, also lead to devastating financial crises.
In brief, anticipating commodity prices is a critical use case, with projections affecting billions of people. These projections are also beneficial for daily commodity professionals such as investors and commodity managers.
Supply constraints, rising costs, inflations, war, pandemics, and logistical issues, along with rising energy demand and geopolitical tensions, have resulted in major changes in the commodity sector in recent months.
The Price Forecasting method by PriceVision is essential reading for managers looking to make informed sourcing decisions to stay ahead of the curve in the commodity price forecasting industry, with independent market intelligence on changes in global supply chains and accurate forecasts for supply price scenarios, as well as supply and demand forecasts and analysis.
Conclusion:
Raw material pricing and demand changes have a substantial influence on the bottom line of manufacturing enterprises. The majority of businesses still depend on gut instinct when making mega-dollar choices. Hence, it’s advisable to rely on Artificial Intelligence and machine learning-based forecasting tools like https://pricevision.ai/ and get the best revenue for the business. To know more about commodities and the market trends on commodity price forecasting check out our blog section pricevision.ai/blog