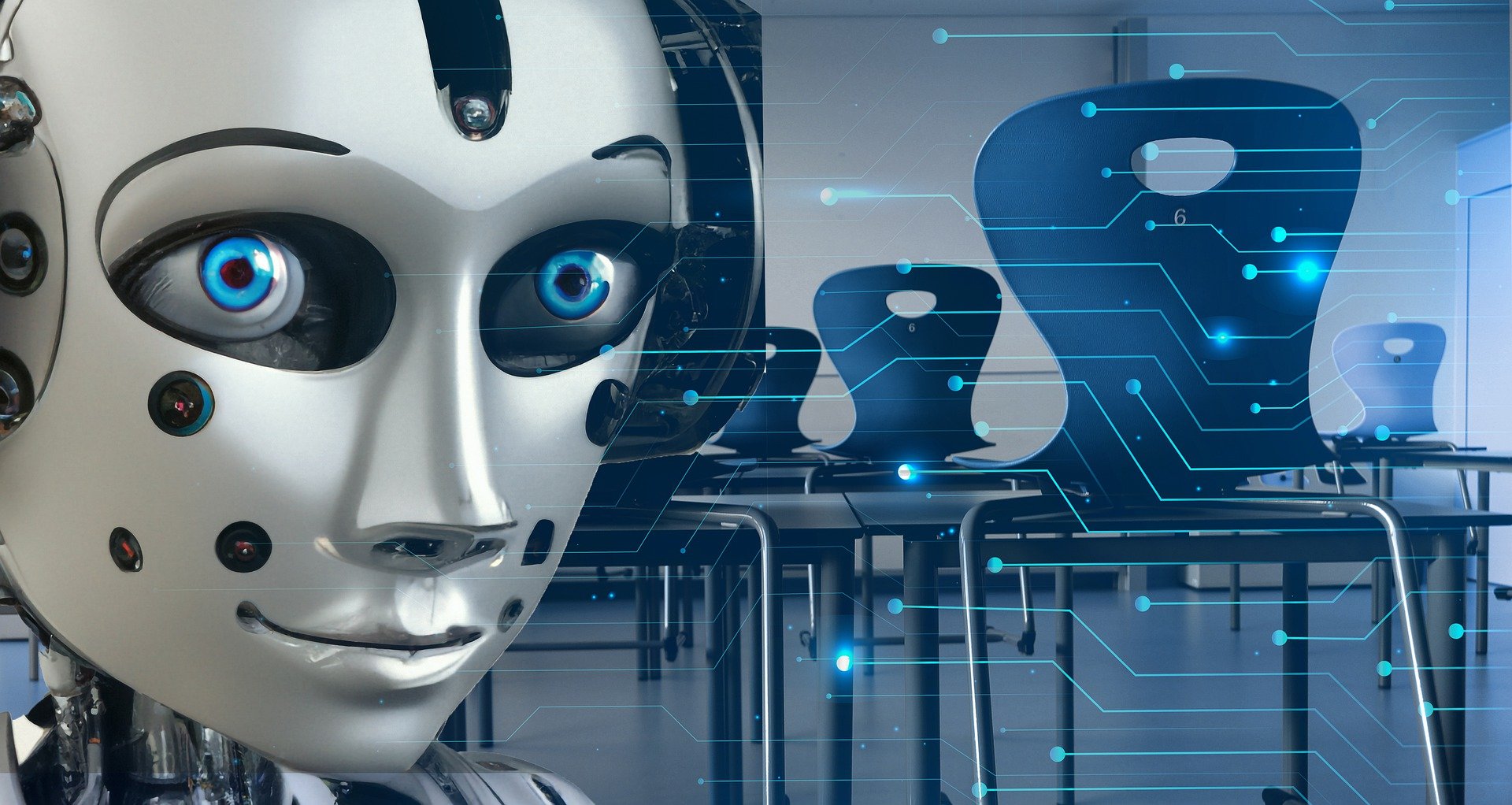
We’re in the midst of a technological revolution and data science is at the centre of much of what we do. From powering Artificial Intelligence (AI) to providing insights into our customers, data science enables us to use large amounts of data quickly and efficiently. For businesses, this means they can make faster, better decisions with more accuracy. It’s no surprise, then, that organizations like ChatGPT are leveraging the power of data science to help them build fast, powerful systems that are incredibly useful for customers.
ChatGPT utilizes data science in many ways, including natural language processing (NLP), knowledge graphs, machine learning and prediction modeling. With NLP, ChatGPT can automatically interpret commands given via voice input or text by using algorithms that understand the meaning behind language. This helps them provide users with faster responses and meaningful conversations – something only possible because of data science.
Knowledge graphs are also a key aspect of how ChatGPT uses data science. Knowledge graphs update quickly with new information so that ChatGPT can give accurate answers to user queries. By utilizing machine learning algorithms on huge datasets in real-time, it allows for an ever-growing Conversational AI (CAI) experience; eventually leading to improved customer satisfaction due to faster response times and better customer service outcomes.
In addition, prediction modeling is crucial for ChatGPT’s CAI system as it helps them identify patterns within user data which leads to more accurate predictions about user behavior in the future – allowing for more personalized experiences with each interaction with a customer.
Challenges Faced by Data Scientists Working with ChatGPT
ChatGPT is an AI powered chatbot platform that promises to deliver personalized conversation experiences. It is built using state-of-the-art data science techniques, such as Machine Learning, Natural Language Processing, and more. That being said, data scientists who work with ChatGPT face a range of challenges to ensure that the service works properly.
Let’s explore some of the major challenges faced by ChatGPT's data science team and how they overcome them in order to create an effective conversational agent.
The first challenge faced by data scientists working with ChatGPT is collecting and pre-processing the necessary training data. To effectively train a Neural Network for natural language processing tasks (such as text understanding), sufficient amounts of high-quality training datasets need to be created and formatted correctly.
Once the training datasets have been gathered, it is important to build a proper testing dataset with varied use cases that covers all possible scenarios. This includes creating suitable conversation flows which account for different user input sequences in order to properly evaluate the performance of the model.
The next challenge is developing an effective ChatGPT model that can generate meaningful responses from user inputs. This involves utilizing various techniques from machine learning such as deep learning architectures and recurrent neural networks that are capable of reasoning over large amounts of data in order to produce accurate results. Additionally, careful consideration must be given when creating conversation logic flows to ensure the bot can handle a range of situations without getting stuck or displaying inadequate responses.
Finally, after the model has been trained and tested thoroughly, it needs to be deployed into production in order for it to be used by users interacting with the chatbot system.