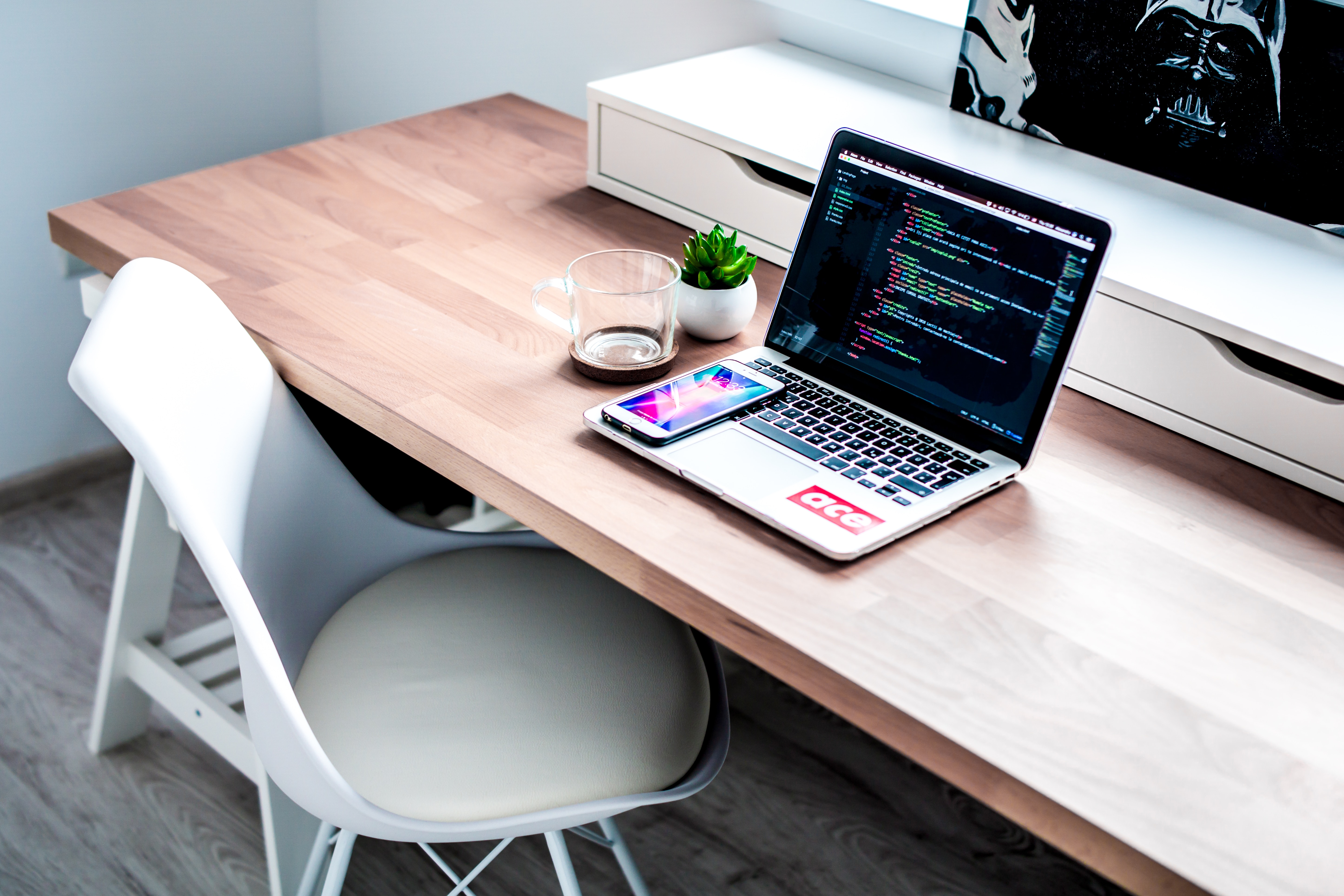
A data science lifecycle describes the iterative way involved in unfolding, delivering, and maintaining any data science product. Because no two data science projects are alike, their life cycles differ as well. Nonetheless, we can imagine a broad lifecycle that includes some of the most common data science steps. The use of machine learning algorithms and statistical practices that result in better prediction models is part of the general data science lifecycle process.
Data extraction, preparation, cleansing, modeling, and evaluation are some of the most common data science steps involved in the entire process. Data Science can be defined as the full operation of gaining applicable perceptivity from raw data, which includes statistical analysis, data analysis, machine learning ways, data modeling, data preprocessing, and so on.
To produce prediction models, data scientists presently use tools similar as AI and complicated machine learning algorithms. The data used for exploration originates from a variety of sources, involving websites, apps, social media, third- party websites, marketing behemoths, and customer support systems
How does FutureAnalytica’s data engineering improve data quality and ensure its accuracy?
It is possible that the data you have will not always be sufficient to build the appropriate AI models. Using data from a variety of sources, FutureAnalytica data enrichment applications can enrich data for the construction of high-end AI models and uncover deep data insights. Data management is a time-consuming IT task. End users can manage data from various sources and integrate it into the platform with the help of FutureAnalytica data management apps, allowing for seamless collaboration and the development of new Artificial Intelligence models.
Why is good data so important?
Businesses could lose a lot of money if bad data is used. Incorrect analytics, incorrect business strategies, and operational difficulties are frequently blamed on bad data. Data quality issues can have a number of negative economic consequences, including increased costs when products are shipped to incorrect customer addresses, missed sales opportunities when customer records are inaccurate or incomplete, and penalties for financial or regulatory compliance reporting errors.
Steps involved in Data science lifecycle for a Data Science Project
1. Define the issue- First; the data problem that needs to be solved must be precisely defined. The issue should be clear, concise, and quantifiable. Many businesses define data problems too broadly, making it difficult, if not impossible, for data scientists to translate them into machine code.
2. Gather information- It’s time to collect data now that the problem has been clearly defined and a suitable approach has been chosen. All collected data, including collection dates and other useful metadata, should be organized in a log.
It is critical to understand that collected data is rarely immediately ready for analysis. The majority of data scientists devote a significant amount of time to data cleaning, which includes removing missing values, identifying duplicate records, and correcting incorrect values.
3. Examine the data- Data analysis comes after data collection and cleanup. At this point, there is a chance that the chosen data science approach will fail. This is to be expected and anticipated. In general, it is recommended to begin by experimenting with all of the basic machine learning models because they have fewer parameters to adjust.
4. Analyze the results- It’s finally time to interpret the results of data analysis. The most important consideration is whether or not the original problem has been resolved. You may discover that your model is functioning properly but producing subpar results. One solution is to add more data and keep retraining the model until you are satisfied with it.
Data Science Applications in Business
1. It improves business predictability.
When a company invests in data structure, it can use what is known as predictive analytics. With the backing of a data scientist, it’s achievable to employ technologies akin to Machine Learning and Artificial Intelligence to work with the organization’s data and, as a result, do more exact anatomies of what’s to come.
2. Provides real-time intelligence
In order to identify their company’s various data sources and develop automated dashboards that continuously scan all of this data, the data scientist can work with RPA professionals.
This information is critical for your company’s operation to make further accurate and timely opinions.
3. Preference for marketing and sales
Based on data nowadays, marketing is a generic phrase. The explanation is simple we can only give results, perspectives, and goods that are genuinely in line with client prospects if we’ve data.
As we have observed, data scientists can combine data from several sources, handing over their crew with further accurate perception. Can you fantasize carrying the full client journey chart, taking into account all of your customer’s relations with your brand? Data Science makes this possible.
As a result, you enhance business productivity and may make opinions now that will have an impact on your company’s future.
4. Enhances data security
One of the advantages of Data Science is the work done in data security. Data scientists, for illustration, work on fraud protection systems to keep your company’s guests safe. On the other hand, he can probe reproducing patterns of actions in a company’s systems in order to descry any design excrescencies.
Conclusion
Visually, it is clear that in data science lifecycle we require more precise data. It entails looking for patterns, and in order to detect patterns, data is required. We will specifically make the assumption that we have access to training data: a bunch of perceptions for which we know the class of every perception.
We hope you found this article informative and helpful in understanding different stages of lifecycle of data science. By automating tasks, our No-Code AI platform improves operational efficiency. Please contact us at info@futureanalytica.com if you have any questions about Data Science, Machine Learning, or AI-based platforms.