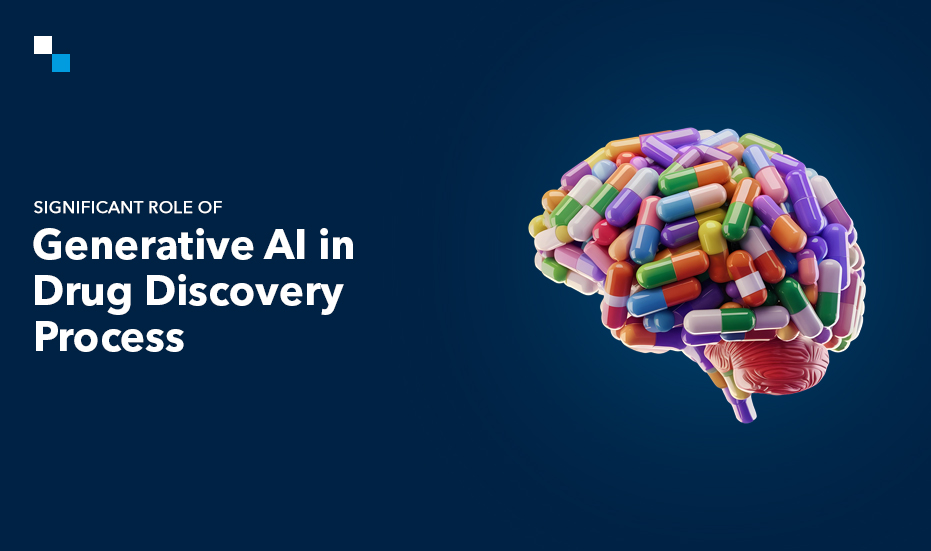
Generative AI has gained significant attention with innovations like ChatGPT, transforming various sectors, including healthcare. Generative AI in drug discovery addresses traditional challenges such as high costs, lengthy development processes, and regulatory hurdles. Generative AI development enables pharma companies to optimize and streamline their drug development stages, which enhances operational efficiency and accelerates growth. In this blog, we highlight the role of gen AI in drug discovery and how it offers a more efficient and effective approach to developing new medications.
Role of Generative AI in Drug Discovery: A Step-by-Step Overview
Step 1: Target Identification and Validation
Generative AI in drug discovery expedites the identification of the correct biological targets, such as proteins or genes responsible for diseases. AI analyzes vast datasets to predict potential disease drivers that help early elimination of ineffective options.
Step 2: Hit Discovery and Molecular Generation
AI generates novel molecular structures with desired properties like high target affinity, solubility, and low toxicity, accelerating hit discovery. With deep learning, AI creates compounds that match specific chemical & biological parameters, which eliminates physical screening.
Step 3: Lead Optimization
Generative AI in drug discovery also automates lead optimization as it can predict how molecular modifications impact efficacy, safety, and pharmacokinetics. It enhances drug bioavailability and stability, enabling faster, more precise optimization of drug candidates.
Step 4: Preclinical Testing
Generative AI uses large datasets to simulate drug performance and enhance preclinical testing. It predicts potential off-target effects and adverse reactions that help companies eliminate unpromising candidates early and prioritize the safest drugs for further development.
Step 5: Clinical Trials
Generative AI analyzes real-world data, patient demographics, and genetics to predict patient response. This allows for better patient stratification, making clinical trials more efficient and safe. Generative AI in drug discovery also identifies biomarkers to monitor trial data in real time and detect early signs of efficacy or safety concerns.
Step 6: Regulatory Submission and Approval
Generative AI automates report generation and analyzes previous submissions to identify potential concerns. Based on the data, the technology predicts regulatory responses, helping companies proactively address issues, accelerating approval, and reducing time-to-market.
Step 7: Post-Market Surveillance
Generative AI in drug discovery also monitors drug safety and efficacy by analyzing data from electronic health records, social media, and patient reports. It detects early signs of adverse events, allowing for timely corrective actions and enhancing drug safety.
Conclusion
The integration of generative AI in drug discovery helps pharma companies streamline their development processes and reap significant benefits. For businesses aiming to stay competitive in the rapidly evolving healthcare sector, adopting generative AI is a strategic move to accelerate growth. Partner with a reputable technology firm for cutting-edge generative AI development services today!