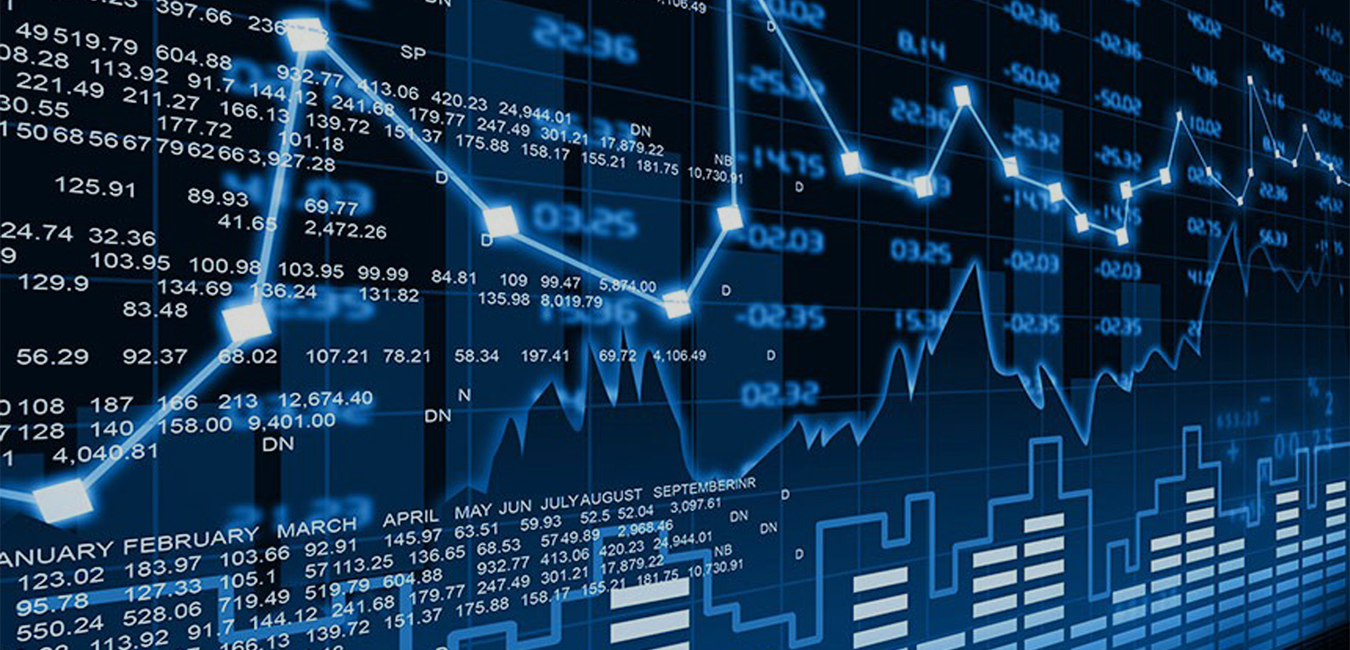
The top two hottest and demanding jobs in the industry are Machine Learning Engineering & Data Scientist in the 21st century. Here, We will know and compare both Machine Learning and Data Science to know what can be the best opportunity for you. Click here to know more information: Machine Learning Engineer Vs Data Scientist
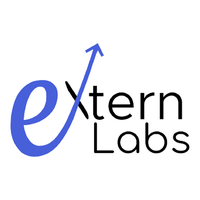
Extern Labs Inc
Related Articles
Rajeev Sharma 2018-11-14
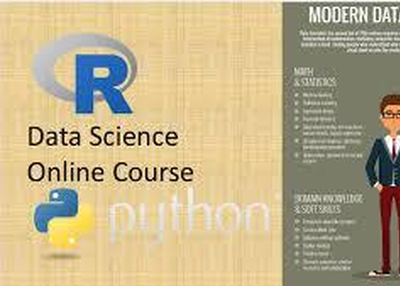
HoningDS.com offers the best online Data Science training. Get trained in Python, R, Statistics and Machine Learning by real time professional. We offer online course for every aspiring Data Scientist in any part of the world. Get hands-on experience using real time projects and become a Data Scientist.
best data science course online
0
Laxman katti 2023-01-25
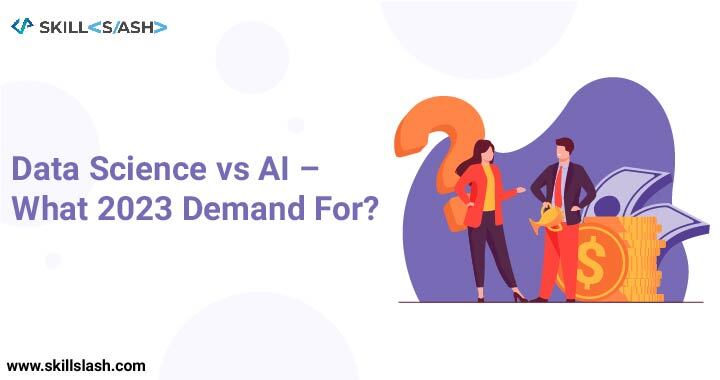
The scope of Data Science is narrower than AI as it mainly deals with data analysis while AI covers a wider range of topics such as robotics, computer vision, and natural language processing. 2023 Demand for Data Science and AIHere are some factors to look at in the demand for both these domains in 2023. Growing Need for Professionals with Skills in Both AreasThe demand for professionals with skills in both data science and AI is growing rapidly. Companies are increasingly looking for individuals who can analyze large amounts of data and use machine learning algorithms to make decisions. ConclusionIt is clear that both Data Science and AI are important fields in the tech industry and will continue to be so in the future.
0
Atul 2023-08-25
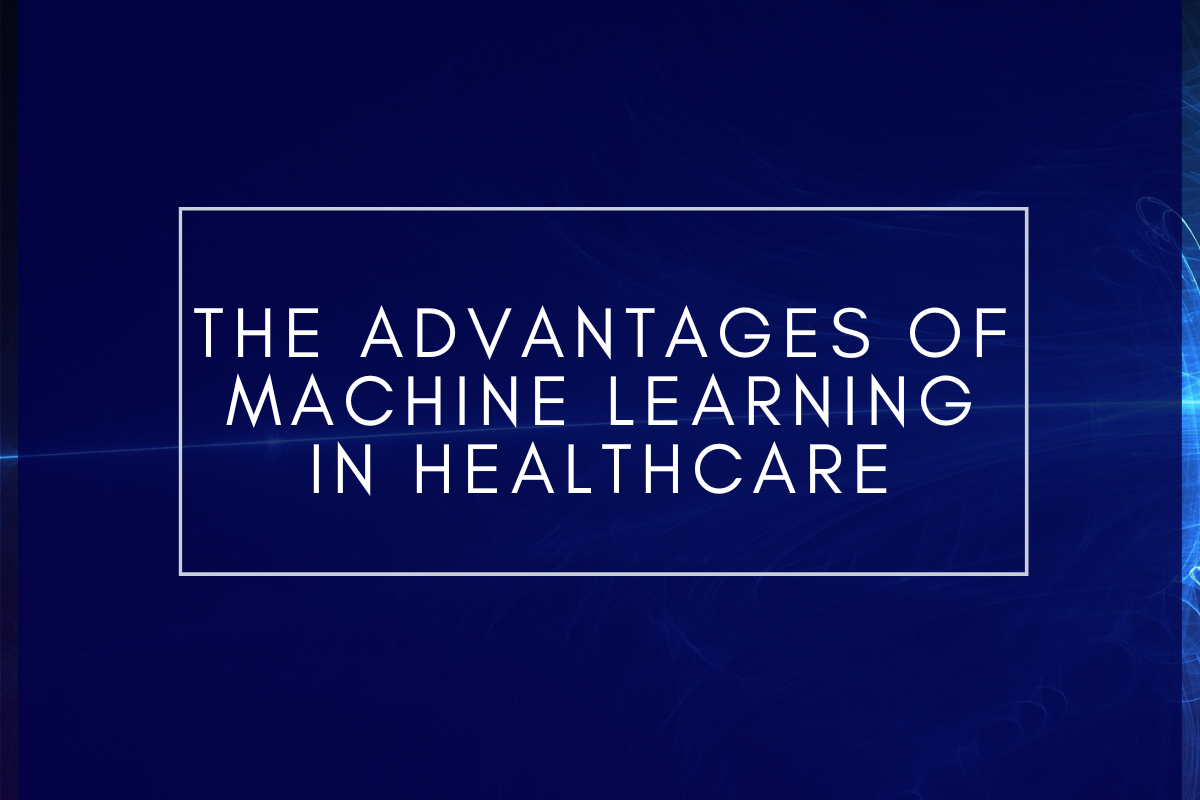
Introduction To Machine LearningWelcome to our blog discussing the advantages of Machine Learning (ML) in the healthcare industry. In addition to improving clinical decision making, machine learning can also help reduce costs and improve efficiency within healthcare organizations. All of these factors can make it difficult for medical professionals to properly utilize machine learning technology for their use. Despite these challenges, the advantages offered by machine learning are hard to deny. Overall, the adoption of machine learning in healthcare has been beneficial despite its associated challenges.
0
Dailya Roy 2023-04-14
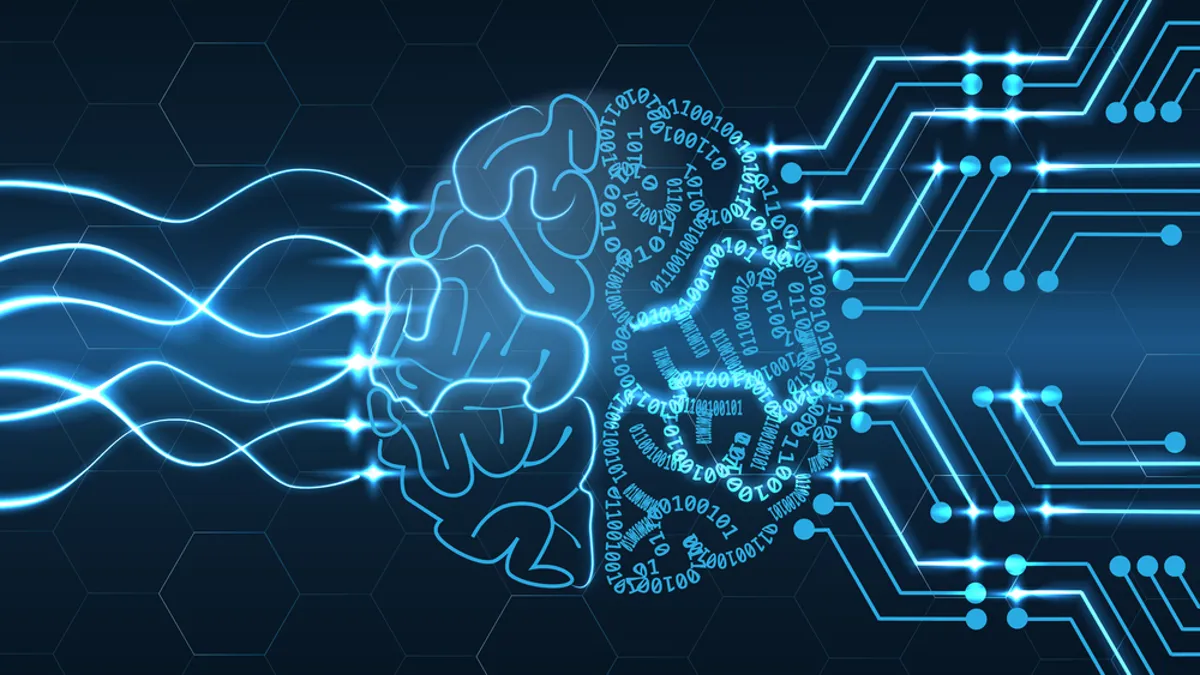
It's a technique for teaching computerized neural networks to detect regularities in data and extrapolate future outcomes based on this analysis. This article is meant to serve as an introduction to deep learning in the context of data science. The Architecture of Deep LearningMany different deep learning architectures see widespread use in data science today. Instances When Deep Learning is UsefulSeveral different areas may benefit from deep learning:The discipline of computer vision has been completely transformed by deep learning. ConclusionIn several areas of data science, deep learning has shown to be a game-changer. It's a technique for teaching computerized neural networks to detect regularities in data and extrapolate future outcomes based on this analysis.
0
jinesh vora 2024-06-25
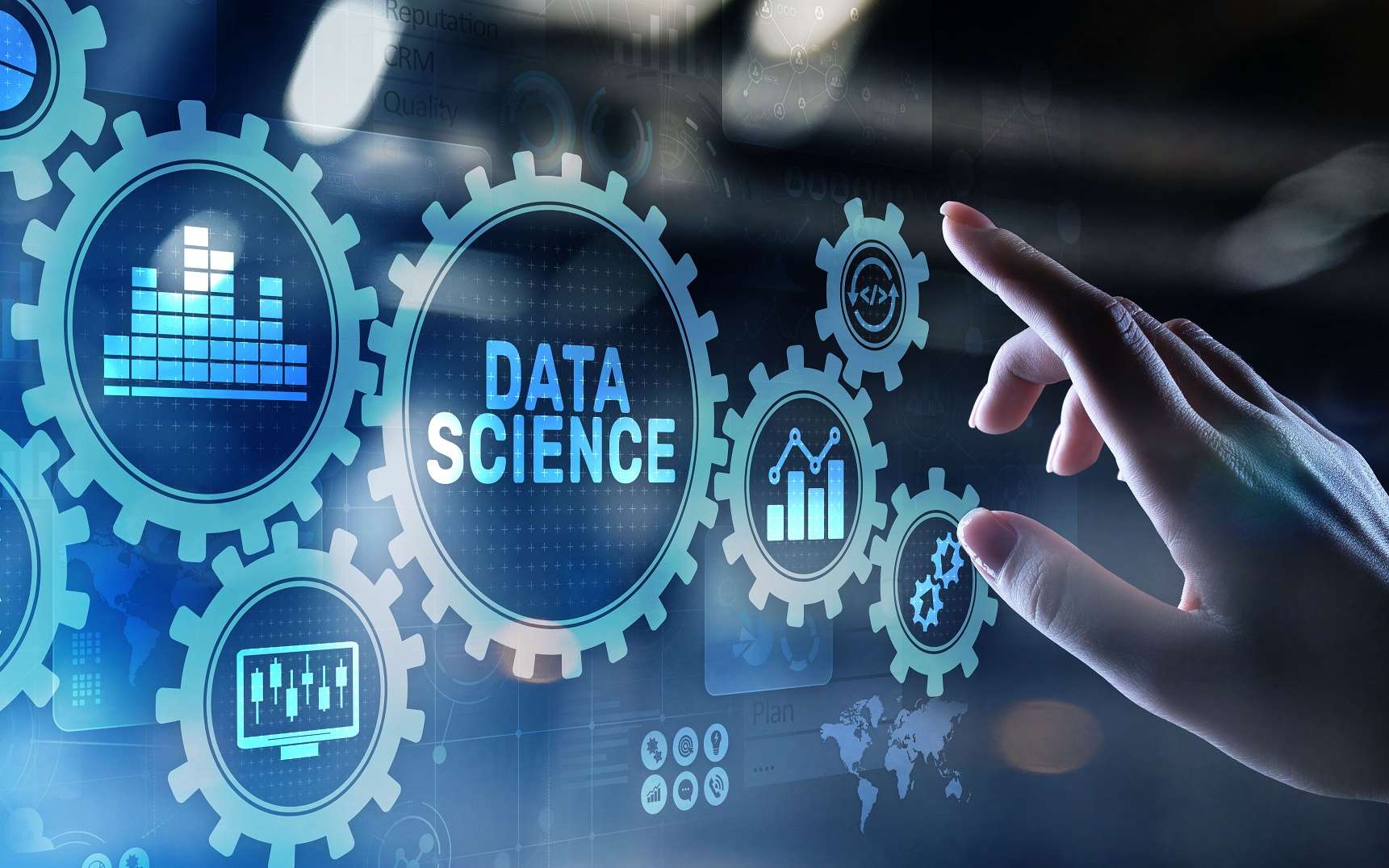
Therefore, a deep appreciation for the complexities of natural language—hopefully—would help a practitioner to navigate the challenges and subtleties of advanced NLP techniques. In addition, combining NER with other NLP techniques, like sentiment analysis, adds even more value to the powerful tool and allows developing more sophisticated, focused applications. It makes a suitable methodology to ascertain the appropriate contextualization of textual data through other NLP techniques, like sentiment analysis and named entity recognition. From named entity recognition and sentiment analysis to text classification and machine translation, advanced NLP techniques change the way one interacts with unstructured data and derives insights from it. The future of NLP in machine learning will probably be fashioned by the sustained development of deep learning and language modeling coupled with the integration of NLP with other cutting-edge technologies, such as knowledge graphs and reinforcement learning.
0
Nishit Agarwal 2023-04-10
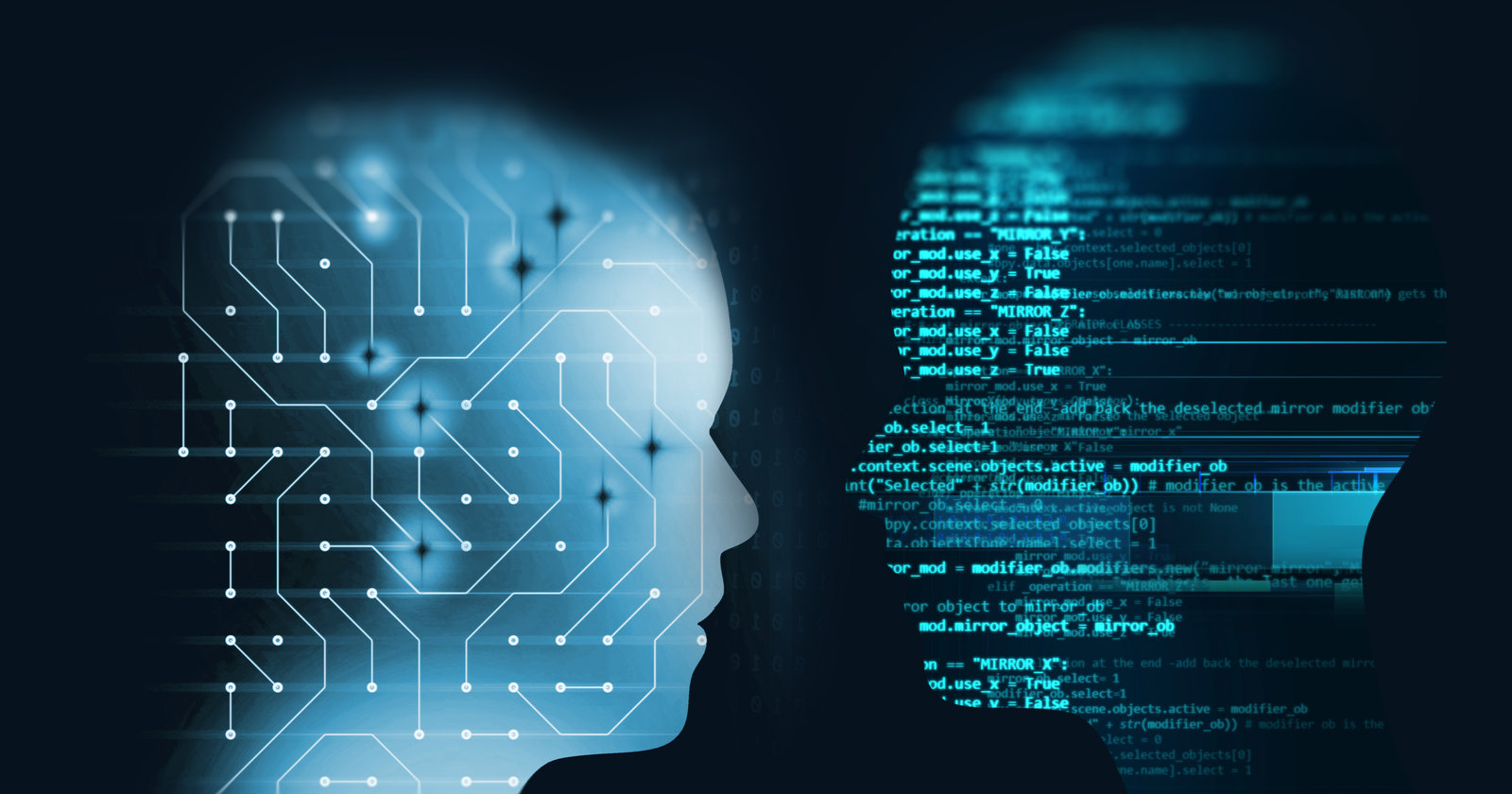
This means that Chebyshev's inequality may overestimate the probability of a large deviation, and caution should be exercised when interpreting the bound it provides. A related limitation of Chebyshev's inequality is that it assumes nothing about the shape or parameters of the probability distribution. In such cases, it may be more appropriate to use a distribution-specific bound rather than relying on Chebyshev's inequality. In such cases, other methods may be more appropriate, such as the Hoeffding's inequality or the Bernstein's inequality. Despite these limitations, Chebyshev's inequality remains a valuable tool in data science and statistics, providing a simple and general way to bound the probability of a deviation from the mean.
0
WHO TO FOLLOW