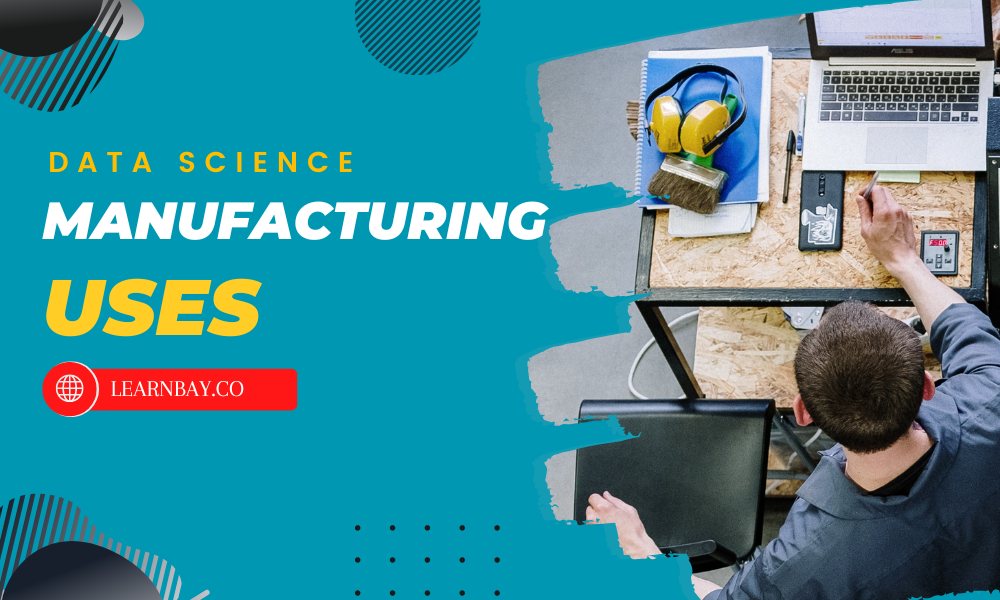
Data science is a multidisciplinary field that includes data management and visualization for all shapes and sizes of data. The study of statistics and probability can provide important insights to manufacturers when enough data are fed into the right data model. Data science is employed in manufacturing for a number of reasons, notably due to a rise in IoT devices relaying productivity and processing data to the cloud.
Data-driven manufacturers will use data science for the following purposes:
- Performance evaluation, quality control, and defect monitoring
- Conditional and predictive maintenance
- Forecasting of throughput and demand
- Supplier relationships and the supply chain
- Pricing on the world market
- Automating processes and creating new facilities
- Innovative methods and materials for improving product development and manufacturing processes
- More sustainability and energy efficiency
Check out the trending data science course in Bangalore if you are considering a career from the ground up.
Top Data Science Applications in Manufacturing
Quality and Performance Data in Real Time
A set of Key Performance Indicators (KPIs) like OEE, or Overall Equipment Effectiveness, can be generated from the machine and operator data. This data makes it possible to conduct a data-driven root-cause study of downtime and scrap.
This establishes the groundwork for a proactive, responsive approach to machine optimization and maintenance, as well as the capacity to react promptly to problems that impact productivity and result in expensive downtime.
Data scientists can then offer a prediction model for machine performance and downtime. These models predict how changes in quality, yield gains, scrap reduction, yield, and, of course, machine downtime will affect the factory floor.
For instance, a manufacturer can concentrate on the main factors affecting performance by graphing Pareto charts on downtime. Manufacturers utilize data science to identify and prioritize the problems that have the biggest effects on production because 20% of causes often account for 80% of downtime.
Monitoring metrics like first-pass yield and scrap counts can help manufacturing organizations uncover innovative methods to reduce costs and boost quality. Operations can be optimized to reduce expensive scrap and rework, whether by testing materials or new procedures.
Fault Prediction and Preventive Maintenance
In today's manufacturing, production may frequently depend on a small number of key components or cells. Data science can be used to evaluate the same data that gives a manufacturer real-time monitoring to enhance asset management and avoid equipment failure. Understanding why a machine fails is the first step toward predicting when it may malfunction.
Process information like temperature and vibration may flag a problem in big data manufacturing before it leads to failure. Condition monitoring can act as an engineer's check engine light by comparing this data to the optimum performance settings recommended by OEMs for specific machines.
This can help engineers identify the need for service and initiate preventative maintenance that could prevent a critical failure later on. The statistical model used to predict failure, and proactively cut downtime is provided by data science. Have a look at the latest machine learning course in Bangalore to gain in-depth knowledge of tools and techniques.
Demand Forecasting and Inventory Management
All manufacturers place a high premium on promptly fulfilling and delivering customer orders. For the purpose of generating demand and delivery estimates, many manufacturers rely on data science. Due to the emergence of just-in-time (JIT) manufacturing, orders are predicated on constrained schedules and supply chains.
Many manufacturers are employing data science to optimize their supply chains, hedge their stocks, and ensure they can fulfill these orders leanly without ordering too much inventory or manufacturing too many products.
Supply Chain Optimization
Supply chain risk management may be difficult. Inputs include gasoline and shipping costs, tariffs, market shortages, pricing changes, local weather, etc. Data science is employed to handle all of the numerous data points. By creating a data science model that predicts market shifts and lowers risk, high expenses may be substituted with savings.
The phrase "value chains" is widely used to refer to supply chains. Assembly lines are supplied with goods through a well-oiled material and part suppliers system. Forecasting is essential in these interactions to ensure that all required parts are obtained, stocked, and ready for assembly. For sectors like electronics, machinery, or auto assembly, faults like late deliveries or stock shortages may be highly expensive.
Price Optimization
Pricing dictates profit, which is determined by what the market will bear for manufacturers employing data science to discover the optimal price. Prices rise and fall. They need to consider the global market for goods and services if they will do this effectively. Smart manufacturers can utilize the same data that drives data-driven supply chain management to predict changes in industry pricing to maximize profit.
Robotics, Automation, and Smart Factory Design
The strong push toward automation calls for significant investment. Data science is used by engineers and systems integrators to choose the best course of action and ensure that this investment will result in significant productivity benefits. Data scientists analyze data to work with engineers to identify the best prospects for cost savings.
To ensure an ROI, manufacturers who spend millions on robotics and other forms of automation use industry 4.0 technologies with assurance.
Digital twinning, which major manufacturers like Siemens back, offers a novel method for designing and optimizing cutting-edge production facilities. Complex data sets and advanced data science are required. The method uses data from the real world to simulate how new machinery and production designs can affect production.
Product Development and Material Design
Design and material selection decisions can be supported by data science. Having data to support your decisions is essential because many contract manufacturers include product development in their list of services.
Data science is used to choose the optimum method of producing a product or material in accordance with the requirements of the customer, particularly by tool and die design and manufacturing to order businesses.
In order to understand consumers and broader market trends and ensure that the given product complies with standards and meets client expectations, manufacturers developing new products for sale also use data science.
Enterprise and Plant-Wide Sustainability
Several manufacturers are establishing challenging objectives to save expenses and conserve energy, including the intricate computations necessary to lower overall carbon emissions. Multinational food producers like Pepsi Co. have prioritized efficiency and sustainability as important components of their long-term plans. They employ data analytics to achieve and surpass these goals by controlling their supply chain and forecasting their own energy consumption.
Conclusion
Thousands of data science positions are already filled, and thousands more are expected in the near future, indicating a promising future for data science in manufacturing. The need for data science to make sense of everything will increase along with the number of smart factories. There are also many pieces of training offered as a result of this demand. So don’t forget to check Learnbay’s data science courses in Bangalore, and familiarize yourself with the newest tools.