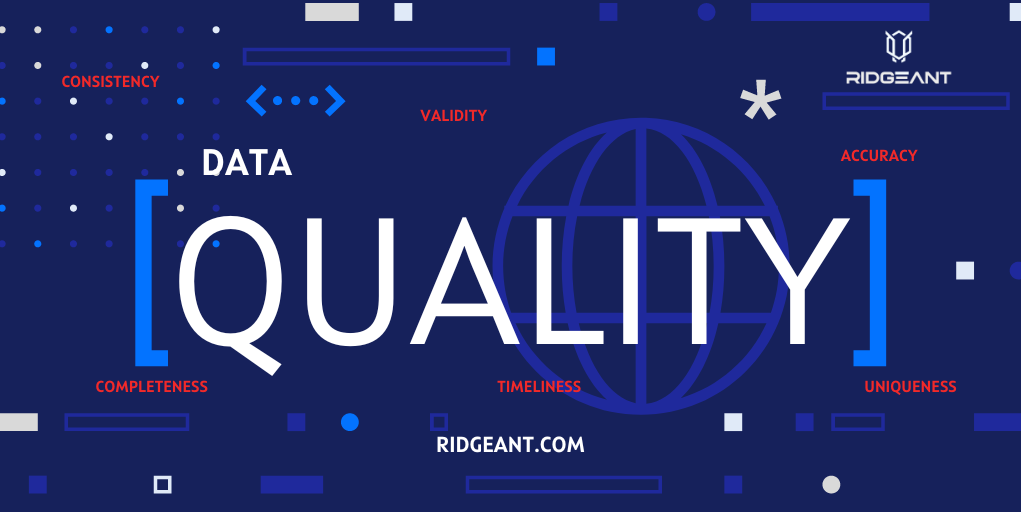
- Wrongly spelled customer name leading to confusion?
- Incomplete information leading to loss of business?
- Lack of emergency information delaying the further course of action?
These are some of the adversities faced when the quality of data is poor. Data quality is an important aspect of a successful and secure business, especially when the entire industry world is being driven by data.
Good quality data can bring wonderful results, newer customers, profitability, and productivity. Bad-quality data can just do the opposite – lessen business results, clients, and effectiveness. There is no option for organizations but to focus on maintaining and monitoring good data quality.
Having good quality data consistently cannot be taken for granted. It needs a well-thought-of mechanism to keep checking for quality, monitor its progress, and deal with adversities as and when they arrive. As easily said than done, with the spread of data going far and wide, the structure of data and its components are widening and increasing in complexity, leading to a tougher task in quality maintenance.
This article attempts to first understand what data quality is, its importance, the key data quality issues that could hamper business, actions to be taken to avoid these issues, and best practices that can be of great assistance to avoid such quality hassles.
What is Data Quality?
Data quality refers to the state of qualitative or quantitative pieces of information. It is generally considered high quality if it is fit for intended uses in operations, decision-making, and planning. It is deemed of high quality if it correctly represents the real-world construct to which it refers. – Wikipedia
Data quality focuses on the competence of a data bulk to offer the organization what it is looking for. It could belong to any industry segment and any phase of work but what is most important is to effectively serve the client’s requirements with the best quality.
With the data horizons growing far and wide, data-related issues are moving beyond just being geometrical or electronic. Now, there are economic, financial, and political influences that can hinder business growth – machine learning issues, and human generation of data being some of them.
It is getting multi-dimensional, adhering to a variety of parameters like documentation, metadata, relevance, context-rich know-how, timelines, etc. Attaining high data quality is now a prime goal for data-enriched companies.
What is now needed is a systematic group of systems and processes that are ingrained in the organizational workflow to adhere to the best quality standards. These steps must stick to business objectives, roles, and responsibilities to avoid quality issues and create a self-driven culture.
Why is Data Quality So Important?
Data quality reaches out to all types and sizes of organizations and industry segments. Here are some standardized reasons why data quality, now, is an indispensable ingredient in the working of business units.
This is what good data quality offers, in creating an enterprise data quality process and thereby, creating a data quality culture:
- Enhanced client experience
- Reliable reporting and analytics
- Increased return on investment
- Optimal operating processes
- Successful modern-day technology plans
- The good quality outcome of the investigation
A Good Read: Data Quality Statistics 2023 – Everything You Need to Know
Major Data Quality Issues in Data Science & Ways to Avoid Them
There are certain significant data quality hassles faced by organizations that must be strictly taken care of, or else it could lead to a disastrous implementation and disturbed workflow. Here are some of the major data quality issues:
Duplication of Data
One of the most common issues organizations face is entering data multiple times leading to duplication. This could happen while data entry or while pulling data out of multi-layered systems to be merged together. Data duplication could lead to inaccurate results.
Manual Errors in Data Entry
Companies face this very routine problem, especially when the data is being entered manually. Humans are bound to make mistakes like typos, missing fields, data entered in wrong fields, etc. while entering data and this could lead to problems while executing IT solutions.
Format Inconsistency
Storing data in incompatible formats is an issue faced by many. The format for each data component must be well-defined based on the nature of the field. A date field must be in a proper date format based on geography rather than just a character field. All calculations must be properly given the number data type, or else it will not give accurate results.
Data Discrepancy
Merely maintaining the format of data isn’t sufficient. You must ensure that the data is stored in a consistent data type with units of measurement attached if any. If the volume is being measured in liters, it cannot be stored in gallons in another field. Computing them together will give inaccurate results.
Errors Committed by Machines/OCR
Many times, when there is bulk data entry, organizations rely on machine-based entry or Optical Character Recognition (OCR) based entry. Images are scanned and text is taken from the scanned data which may not be perfect, leading to misconceptions. It is tough to extract the useful part of data from the machine-based output.
Blunders While Transforming Data
There are situations when data is transformed and loaded from one data type to another, for e.g., from MS Excel to a database. There are chances of uncertainties while transforming data from one type to another.
Security Hassles
It is a must that any type of data that is being monitored, transformed, or operated upon must follow the rules and regulations of the organization or standard ones like HIPAA, PCI DSS, etc. Not complying with these standards can incur a lot of overhead in terms of fines or lack of customer interest. The absence of data quality training programs and integrated data management can lead to a loss of customer quality and trust.
Not Catering to Hidden Data
Many times, companies fail to capture and extract the hidden data that is much valuable for customer insights. It is this hidden data that can offer a detailed and insightful view of business information and help capture a better client segment. But the problem arises when organizations keep focusing on the outer layer of data that is superficial and tend to neglect the big iceberg of data within.
Irrelevant Data and Data Definitions
While moving through the pools of data, there are chances of coming across data that is irrelevant and does not adhere to the basic database principles. Also, the definition of data components must be kept consistent through the databases at different locations and systems. Only then can a smooth transfer of data can happen within systems, based on standard norms.
Unreliable Keys and Data Integrity
Data is linked to primary keys and foreign keys. With data transformation and aggregation, there are chances of mismatched keys, leading to referential integrity issues. Data profiling may have to be done to make the entire data pattern systematic and integrated. Sometimes, data is locked in warehouses that are not easily accessible. In such cases, it is difficult to avail data with the utmost integrity.
Key Best Practices to Solve Data Quality Issues?
Above mentioned are some of the most common data quality issues faced by organizations and below mentioned are some of the key measures that can be taken to avoid these hassles:
- Focus firstly on cleaning the original source of data
- Apply precision identity or entity resolution to data
- Create metadata layers for common business and data definitions
- Leverage data profiling to measure data integrity and data frequency
- Generate insightful data quality reports and dashboards
- Create issues logs and threshold values for alerts and notifications
- Understand data completely based on business needs
- Normalize your data through modern tools and technologies
- Give attention to training and ensuing a data-driven culture
- Apply regular data checks for duplication, consistency, security, validation, formatting, integrity
- Make use of statistical techniques like regression analysis, hypothesis testing, Statistical Process Control (SPC)
Conclusion
These were some of the major data quality issues that any and every organization could face, be it any industry segment or domain. As we go along offering the best of data analytics to a wide range of customers around the globe, ensuring good data quality is key. We have a well-prepared set of policies and standards that are instrumental in keeping a good quality level as far as data is concerned.
If you face any kind of hassle in maintaining and monitoring the data quality, reach out to us. Our data excellence experts will offer a flexible and personalized plan that can easily help you garner the best of data quality.
Note: This Post Was First Published On https://ridgeant.com/blogs/data-quality-issues/