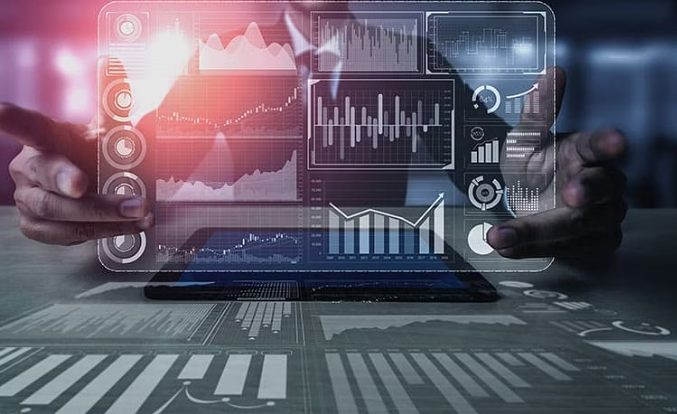
Introduction
When it comes to managing and analyzing vast amounts of data, having the right data engineering platform is crucial. Whether you are a small business looking to streamline your data processes or a large enterprise in need of advanced analytics capabilities, choosing the best data engineering platform can make all the difference. With the rise of data-driven decision-making and the increasing importance of data in today's business landscape, the demand for data engineering consulting services has never been higher. In this comparative guide, we will explore the top data engineering platforms in 2024 and beyond, highlighting key features, capabilities, and trends to help you make an informed decision for your data engineering needs.
Overview of Leading Data Engineering Platforms in 2024
As we step into 2024, the diversity and capabilities of data engineering platforms continue to evolve, offering tailored solutions for businesses of all sizes. Key players in the market include Apache Spark, renowned for its robust in-memory data processing; Google Cloud Dataflow, offering scalable serverless data operations; Amazon EMR, a versatile platform for big data processing; Microsoft Azure Data Factory, known for its comprehensive data integration services; and Snowflake, celebrated for its ease of data sharing and analytics. Each platform brings distinct advantages, from high scalability and real-time processing to extensive integration options with leading data analytics tools.
For businesses embarking on or expanding their data engineering journey, the choice of platform should align with specific needs regarding data handling, security protocols, and integration with existing systems. These platforms not only present diverse pricing models but also adapt to emerging data engineering trends, ensuring that businesses can find a solution that scales with their growth and data strategy ambitions.
Key Features to Consider When Choosing a Data Engineering Platform
Selecting the ideal data engineering platform requires a nuanced understanding of several pivotal features that align with your project's unique requirements. Essential elements to scrutinize include the platform's capability for seamless data integration, ensuring smooth workflow between various data sources and systems. Scalability is another critical feature; the platform must be able to grow alongside your business, handling increased data volumes without compromising performance. Real-time processing capabilities are essential for businesses needing instant data analysis for timely decision-making.
Additionally, the utility of data transformation tools cannot be overstated, as they facilitate the conversion of raw data into a more valuable form, ready for analysis. Support for multiple data formats is also vital, ensuring flexibility and compatibility with different types of data. Lastly, the ease of integrating the chosen platform with existing data analytics tools and services should be considered, guaranteeing a cohesive and efficient data engineering ecosystem. These features collectively determine the platform's ability to meet specific data processing requirements and support the extraction of actionable insights.
Comparative Analysis of Data Storage and Processing Capabilities
When diving into the nuances of data storage and processing capabilities among top contenders, it becomes apparent that each platform has carved out its niche. Apache Spark distinguishes itself with superior in-memory processing prowess, adept at handling massive datasets swiftly, making it a prime choice for real-time analytics. Google Cloud Dataflow, conversely, shines with its serverless architecture, offering elasticity that dynamically adjusts to workload variations, ensuring efficient data handling without the need for constant infrastructure management. Amazon EMR stands out for its flexibility in processing vast amounts of data, leveraging the robust AWS ecosystem to provide a scalable solution for both batch and stream processing tasks.
Microsoft Azure Data Factory and Snowflake also bring unique strengths to the table; Azure excels in seamless data integration across diverse environments, while Snowflake's architecture allows for instant scalability and collaborative data sharing without compromising on performance. When considering these platforms, it's vital to weigh the specifics of data storage flexibility, the agility of processing speeds, and the ability to maintain performance at scale to find the best fit for your data engineering requirements.
Evaluating Data Security and Compliance Measures Across Platforms
Evaluating the security frameworks and compliance standards of data engineering platforms is a critical step in safeguarding data integrity and adhering to regulatory requirements. Amazon EMR, with its comprehensive security measures such as encryption methods for data at rest and in transit, alongside AWS Key Management Service integration, showcases a robust approach to secure data management. Microsoft Azure Data Factory further exemplifies the importance of compliance and data governance by offering advanced features like data encryption, masking, and classification to align with regulatory standards. It's imperative to meticulously assess the security features, including encryption standards, access controls, and audit capabilities of each platform.
Additionally, understanding how these platforms align with global compliance mandates such as GDPR, HIPAA, and CCPA ensures that the chosen solution not only protects sensitive data but also adheres to legal and regulatory frameworks. This comparison aids in identifying a platform that not only meets the technical and functional requirements but also ensures the highest levels of data security and compliance.
Usability and User Experience: Which Platform Stands Out?
In the realm of data engineering platforms, the ease of navigation and efficiency in executing data processes significantly impacts user satisfaction and operational productivity. Snowflake emerges as a frontrunner with its streamlined interface and straightforward integration capabilities with analytics powerhouses like Tableau and Looker, facilitating a frictionless experience for both seasoned data professionals and those new to data engineering.
On the other hand, Google Cloud Dataflow garners appreciation for its visual programming environment and accessible interface, which demystifies complex data workflows for developers and engineers alike. Aspects such as the initial learning curve, available documentation, and the quality of customer support are crucial in determining the overall user experience. Platforms that offer comprehensive educational resources and responsive support teams tend to foster a more productive environment, enabling teams to leverage the full potential of the platform's capabilities. The emphasis on user-centric design and supportive resources across these platforms highlights their commitment to not just meeting but exceeding the usability expectations of the modern data engineer.
Pricing Models and Cost-Efficiency of Data Engineering Platforms
Understanding the financial implications of deploying a data engineering platform is paramount for businesses operating within a budget. Apache Spark, an open-source framework, presents an appealing option for those looking to minimize upfront costs, relying instead on community support for advancements and troubleshooting. Google Cloud Dataflow and Amazon EMR adopt a usage-based pricing strategy, offering flexibility that aligns operational expenses directly with data processing demands. This model is particularly beneficial for projects with fluctuating workloads, as it allows for cost adjustments in real-time.
Microsoft Azure Data Factory's tiered approach to pricing provides businesses with predictable costs based on their data integration needs, enabling more precise budget planning. It's important for organizations to carefully assess each platform's pricing structure against their data processing volume and complexity to identify the most cost-effective solution. Consideration of potential hidden fees, such as data transfer charges or premium support services, is also crucial in estimating the overall investment in data engineering services.
Future Trends and Predictions for Data Engineering Platforms Beyond 2024
The horizon for data engineering platforms beyond 2024 is set to be marked by transformative innovations. Among the anticipated advancements, the integration of augmented analytics stands out, utilizing AI to enhance data analytics and make the data more accessible to non-expert users. This democratization of data is expected to empower a wider range of professionals to engage with data science tasks without deep technical expertise. Additionally, we foresee a significant shift towards serverless architectures, reducing the operational burden on data teams by abstracting away the infrastructure management.
This will allow teams to focus more on data insights rather than on managing servers. Another emerging trend is the increased emphasis on real-time data streaming services, enabling businesses to process and analyze data as it arrives, thereby making faster decisions. The proliferation of edge computing is also anticipated to influence data engineering platforms, bringing data processing closer to the source of data generation and reducing latency. These trends collectively suggest a future where data engineering platforms are more intelligent, agile, and user-friendly, facilitating a more seamless interaction between businesses and their data ecosystems.
Conclusion
Navigating the intricate landscape of data engineering platforms requires a discerning eye for features that resonate with your organizational goals and data strategy. This guide has endeavored to shed light on premier platforms like Apache Spark, Google Cloud Dataflow, Amazon EMR, Microsoft Azure Data Factory, and Snowflake, underscoring their unique strengths in handling, processing, and securing data. As you deliberate on the optimal choice, weigh considerations such as integration capabilities, scalability, and cost against your specific requirements.
It's also prudent to stay abreast of evolving trends that promise to shape the future of data engineering, ensuring your selected platform can adapt and thrive in the changing digital ecosystem. Remember, the right platform is more than just a tool; it's a catalyst for insight, innovation, and competitive edge. Engaging with data engineering consulting services or bringing on board expert data scientists can further unlock the potential of your chosen solution, propelling your business towards data-driven excellence.