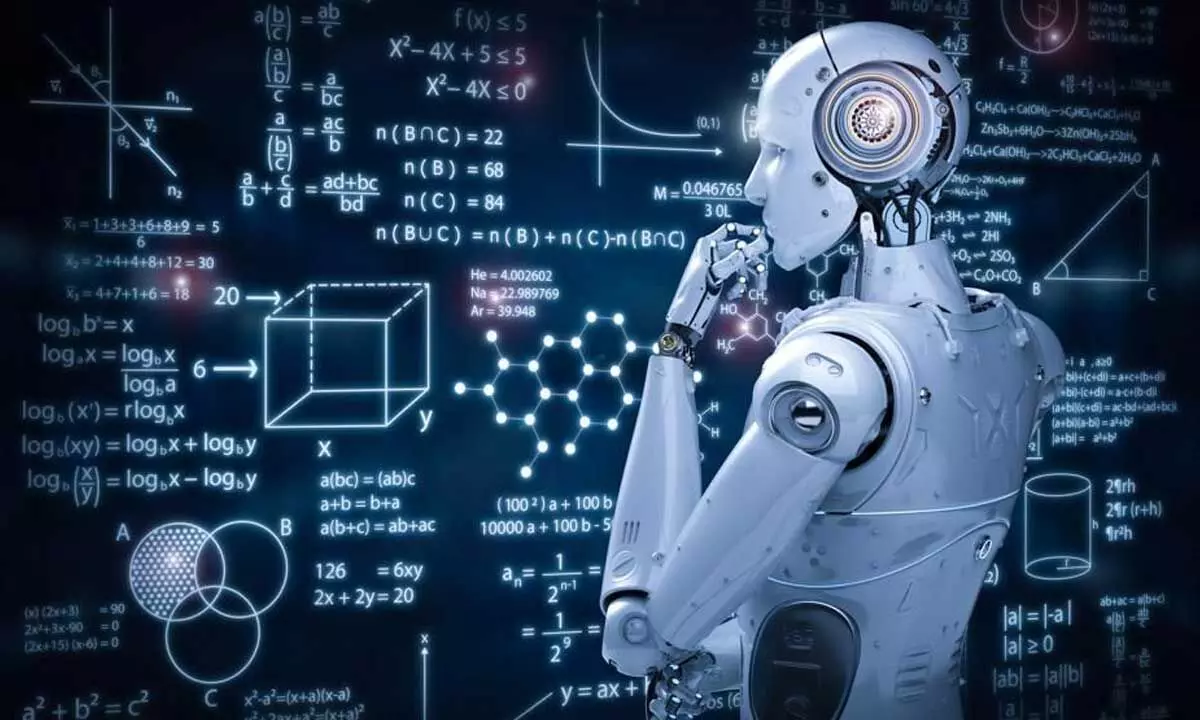
A theorem in statistics and probability theory, "the law of large numbers" defines what happens when an experiment is repeated several times. The big numbers theorem argues that the average of the outcomes of the trials must be close to the anticipated value if the same experiment or research is performed independently a large number of times.
As more tests are run, the outcome converges on the theoretical maximum. The rule of big numbers is a basic idea in statistics, explaining why repeated random occurrences may sometimes produce predictable patterns over time. The theory only applies to large numbers of trials, therefore the average of findings from a few repetitions of the experiment may deviate significantly from the predicted value. However, the reliability of the average result improves as the number of trials grows.
The best online data science courses can enhance your knowledge.
The Law of Large Numbers (LLN) is a staple of probability theory and has significant applications in the field of data science. It's predicated on the fact that the sample mean of a random variable tends to approach the anticipated value of the variable as the sample size increases. The relevance of the Law of Large Numbers in data science will be discussed, along with its foundations.
The Law of Large Numbers in Probability Theory
In probability theory, the idea of the Law of Large Numbers is introduced. It is a basic theorem that the sample mean of a random variable will become closer to the anticipated value of the variable as the size of the sample grows. In mathematical form, this may be expressed as:
Consider a set of n independent, identically distributed random variables X1, X2, X3,...,Xn with mean and variance. Consider the sample mean of the first n variables to be S_n = (X1 + X2 +... + Xn)/n. This means that for every that is positive,
Infinitely small P(|S_n - mu| > epsilon) = 0.
In other words, the likelihood of the sample mean deviating far from the predicted value decreases as n becomes greater.
Data Science and the Power of Large Samples
Many statistical methodologies and procedures in data science rely on the Law of Large Numbers. It's what statisticians use to build solid inferences from data and precise forecasts on their networks.
In data science, sampling is one of the most crucial uses of the Law of Large Numbers. Sampling is a method for estimating a population characteristic by drawing a representative sample from the whole data set. To get an idea of the average height of a country's population, we may, for instance, choose a random sample of people and measure their heights. With a bigger sample, the sample mean will go closer to the actual population average because of the Law of Large Numbers.
Hypothesis testing, a method used to ascertain the truth or falsity of a hypothesis regarding a population, also makes use of the Law of Large Numbers. The goal of hypothesis testing is to establish whether or not a certain hypothesis is supported by data by collecting and analyzing a representative sample of the data. There is less of a chance of committing a type I mistake (incorrectly rejecting a true hypothesis) or a type II error (incorrectly accepting a false hypothesis) when there are more people in the sample.
The Theorem of Central Limits
Central Limit Theorem (CLT), like the Law of Large Numbers, is a cornerstone of probability theory. According to the CLT, regardless of the true distribution of the population at large, the distribution of the sample mean will tend toward normality as the sample size increases. This is an important finding because it enables us to extrapolate from the data we collect to the parameters of the whole population.
The CLT has practical use in data science because it enables us to draw inferences about the sample mean and other sample statistics with a high degree of confidence. Using the sample mean and standard deviation, for instance, we may determine a confidence interval for the population mean using the CLT. Hypothesis tests based on the t-distribution or the z-distribution may also be conducted with the help of the CLT.
Conclusion
The Law of Large Numbers is a staple of probability theory and has significant applications in the field of data science. It makes certain that the sample mean becomes closer to the predicted value of the variable as the sample size grows. This paves the way for solid inferences to be drawn from data and for precise predictions to be made using statistical models. Probabilistic inferences from sample statistics are made possible by the Central Limit Theorem, which has strong ties to the Law of Large Numbers. Together, these ideas provide the backbone of several statistical techniques.
A data science course in India can be helpful to get a better understanding of this subject.