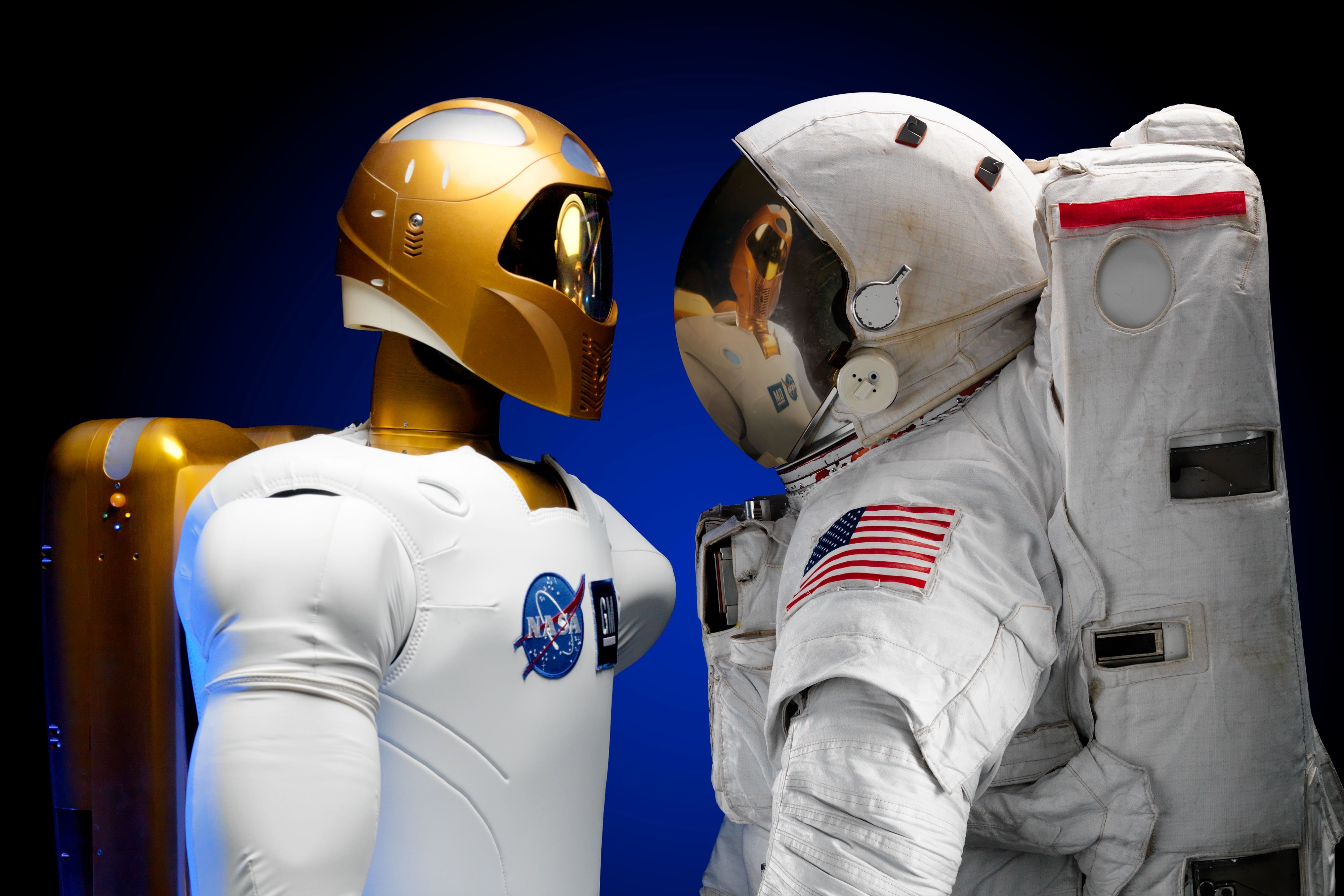
Deep learning, as a subfield of artificial intelligence, has played a key role in some of today's most transformative innovations. Self-driving cars, facial recognition, and translation apps are just a few examples of consumer-facing deep learning applications already accessible.
Data from online retailers is a gold mine for artificial intelligence and deep learning in the retail and e-commerce industries. Each data point contributes to a comprehensive understanding of client behavior. The most successful e-commerce organizations use not only data science fundamentals, but also deep learning approaches to harness this data. Business-critical predictions, such as whether or not a consumer would buy again, can be made using deep learning and artificial intelligence. Deep learning techniques can be used by any company as long as they have access to a big amount of data and they invest in the infrastructure as well as the people who can interpret the data.
We can analyze purchasing intent quantitatively using data science and deep learning techniques. Purchase intent, in mathematical terms, is the likelihood that a customer will buy a product or service. We can construct deep learning models that show with near certainty whether a buyer will buy our product using a mathematical representation of purchase intent and enough data points about our customers. What's even more fascinating is that deep learning algorithms may make a unique prediction for each consumer. If you use business intelligence dashboards or other common data analysis tools, you will only obtain a broad view, not individual predictions. Such information might then be used in a variety of ways, including marketing. We could use higher discounts to entice some consumers, or more features to sway others, and so on. This type of information also aids marketers in determining how best to spend their advertising money.
Ways deep learning predicts your repeat customer
Feature engineering
Data scientists build a set of attributes (input features) that indicate diverse behavior patterns connected to client involvement with a service or product during the process. In a broad sense, features are observable qualities that an ML model considers when predicting outcomes. The goal of feature extraction is to reduce the number of attributes by retaining those that offer the most discriminative information. Feature extraction reduces data dimensionality and eliminates irrelevant data.
Segmentation of customers
Customers are typically segmented by growing companies and those increasing their product ranges utilizing previously defined and selected attributes. Customers can be segmented depending on their lifecycle stage, needs, solutions used, level of participation, monetary value, or basic information. Because every client segment has similar behavior patterns, it's possible to improve forecast accuracy by using machine learning models trained on datasets representing each segment.
Testing and modelling
Its major purpose is to create a prediction model. Specialists frequently train a number of models, tune, assess, and test them to find the one that accurately detects probable data. Data scientists frequently use the performance of a baseline model as a criterion to compare the accuracy of more complicated algorithms.
Some notable prediction models are:
Logistic regression: A binary classification problem is solved using the logistic regression algorithm. It assesses the link between a dependent variable and one or more independent variables in order to forecast the likelihood of an event (features). It actually forecasts the likelihood of a data point falling into the default category.
Decision tree: It is a form of supervised learning method (with a predefined target variable.) While it is most commonly used for classification jobs, it can also handle numeric data. To create a prediction, this method divides a data sample into two or more homogeneous sets based on the most significant differentiator in input variables. A component of a tree is formed with each split. As a result, a tree is formed, including decision nodes and leaf nodes (decisions or classifications).
Random forest: It is an ensemble learning technique that employs a large number of decision trees to improve prediction accuracy and model stability. This is used to solve both regression and classification problems. Based on attributes, each tree classifies a data instance (or votes for its class), and the forest selects the classification with the highest votes. In regression tasks, the average of the decisions of several trees is used.
Monitoring and deployment
Machine learning and artificial intelligence are used to predict client attrition in an ongoing process that never stops. When customer-facing teams provide input or new data becomes available, it monitors model performance and updates features as needed to improve accuracy. The frequency with which a model's performance is evaluated is determined by how quickly data in an organization gets obsolete.